Artificial intelligence has been taking unprecedented strides, especially in the realm of generative models. Today, we present a breakthrough in this field: Composable Diffusion (CoDi). The innovative brainchild of a team from the University of North Carolina at Chapel Hill and Microsoft Azure Cognitive Services Research, CoDi is an AI model capable of generating any mix of output modalities, such as language, image, video, or audio, from any combination of input modalities.
Demo:
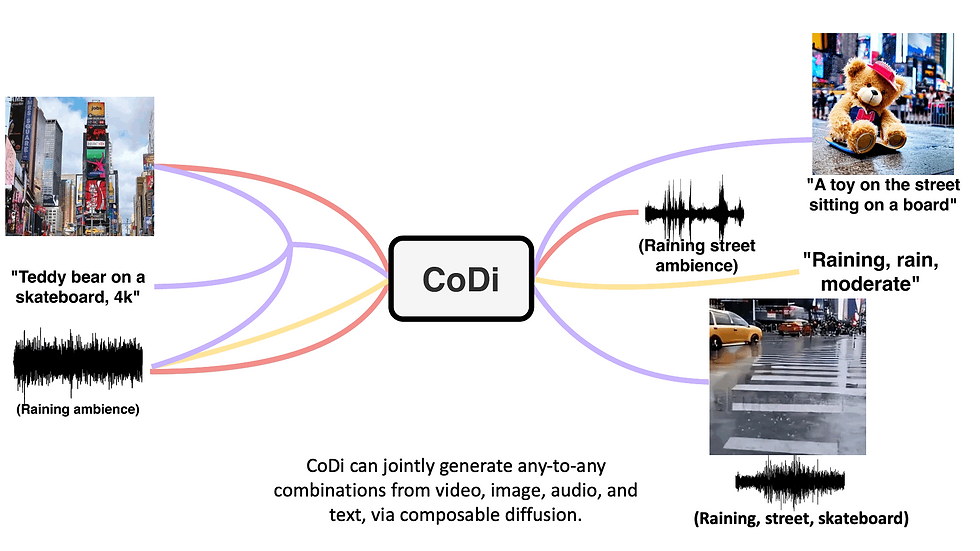
A New Age of Generative AI with CoDi
Unlike existing generative AI systems, CoDi's versatility lies in its ability to generate multiple modalities in parallel, without being confined to a subset of modalities such as text or image for input. It brings a fresh perspective to the generative process, allowing for flexibility unheard of in the current AI landscape.
The unique feature of CoDi is its ability to generate any combination of modalities even when they are not present in the training data. This is achieved by aligning modalities in both the input and output space. Such an approach enables CoDi to freely condition on any input combination and create any group of modalities.
Novel Composable Generation Strategy
CoDi relies on a novel composable generation strategy, bridging alignment in the diffusion process to build a shared multimodal space. This model architecture facilitates the synchronized generation of intertwined modalities, such as temporally aligned video and audio.
In simpler terms, CoDi uses a unique method to analyze different types of input (like text, images, audio, and video) simultaneously. It then uses this analysis to generate a variety of outputs across different formats, all while maintaining the inherent alignment and relationships between these different modalities.
Model Architecture and Training
CoDi employs a multi-stage training scheme, allowing it to be trained on only a linear number of tasks while inferring on all combinations of input and output modalities. This efficient architecture sets the stage for the system's flexibility and adaptability, a significant leap from the restrictions of traditional generative AI models.
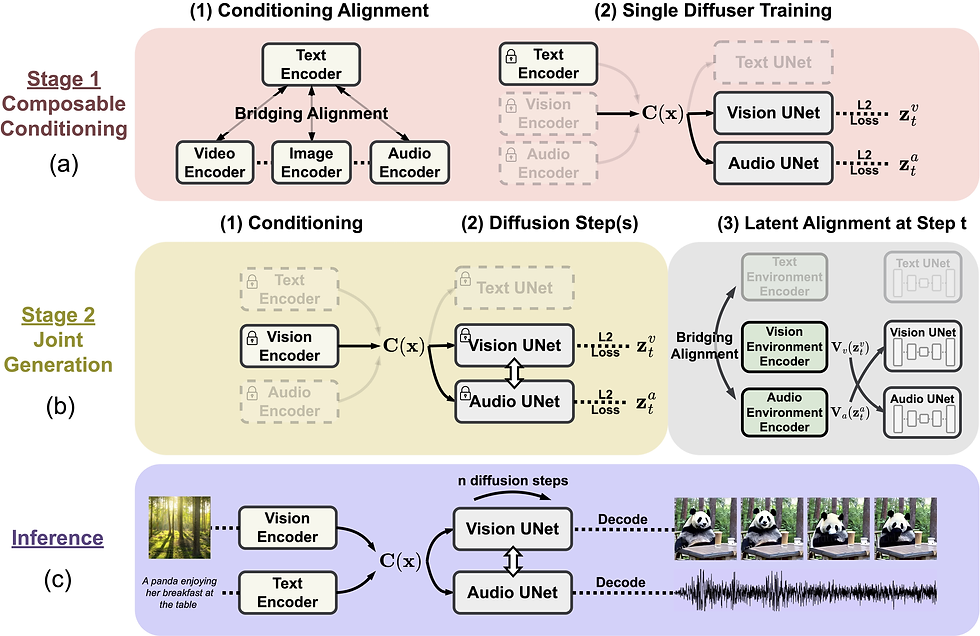
Performance and Future Implications
Highly customizable and flexible, CoDi showcases strong joint-modality generation quality. It either outperforms or is on par with the state-of-the-art unimodal systems for single-modality synthesis, establishing it as a game-changing contender in the AI generative model landscape.
Github: https://codi-gen.github.io/
The introduction of CoDi brings forth a new age of multi-modal AI generation, opening up endless possibilities for the use of AI in areas like content creation, virtual reality, and beyond. With the capability to freely condition and generate any group of modalities, CoDi holds the potential to redefine the future of AI generative models. The world is keenly watching as CoDi paves the way for an innovative future in generative AI.
Comments