What is the Phi-3-mini model?
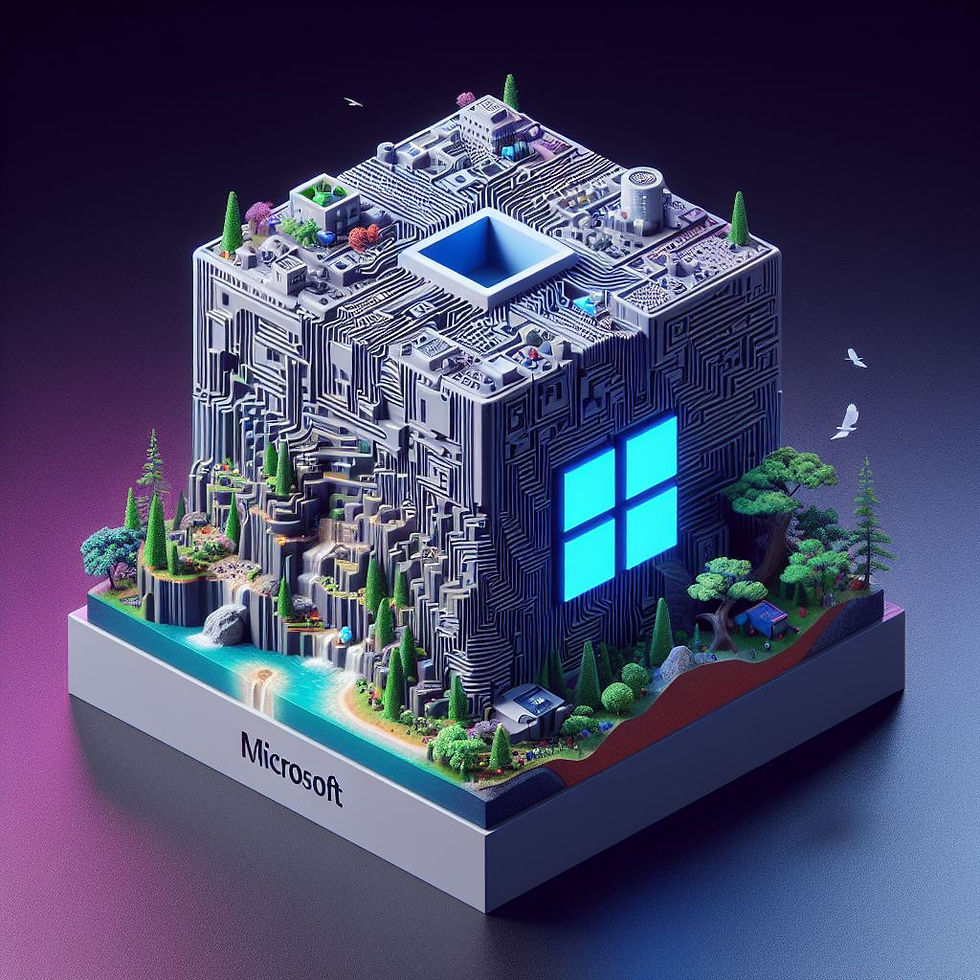
. With 3.8 billion parameters, it's a lightweight yet powerful option trained with both synthetic data and filtered publicly available website data.
What are the key features of the Phi-3-mini model?
The Phi-3-mini model is a dense decoder-only Transformer model optimized for ONNX Runtime with support for Windows DirectML, making it compatible across various hardware platforms, including GPUs, CPUs, and mobile devices. It's available in two context-length variants, 4K and 128K tokens, and is instruction-tuned to follow different types of instructions, mirroring human communication patterns.
How does the Phi-3-mini model ensure safety and alignment with human preferences?
This model undergoes a post-training process that includes supervised fine-tuning and direct preference optimization, ensuring precise instruction adherence and robust safety measures. These steps align the model with human preferences and safety guidelines, making it suitable for a wide range of applications.
What are the performance benchmarks of the Phi-3-mini model?
Assessed against benchmarks testing common sense, language understanding, math, code, long context, and logical reasoning, the Phi-3-mini model showcases robust and state-of-the-art performance among models with less than 13 billion parameters. This performance makes it suitable for various tasks requiring advanced language comprehension and reasoning abilities.
What are the intended use cases for the Phi-3-mini model?
Designed to accelerate research on language and multimodal models, the Phi-3-mini model serves as a building block for generative AI-powered features. It's suitable for both commercial and research use in English, particularly in applications requiring memory/compute-constrained environments, latency-bound scenarios, strong reasoning (especially in math and logic), and long context.
What considerations should developers keep in mind when using the Phi-3 model?
While the Phi-3 model offers advanced capabilities, developers should consider common limitations of language models and evaluate and mitigate for accuracy, safety, and fairness before deploying it for specific downstream use cases, especially for high-risk scenarios. Adherence to applicable laws and regulations, including privacy and trade compliance laws, is essential for responsible usage.
Alternatives to the Phi-3-mini model
OpenAI's GPT series: OpenAI's Generative Pre-trained Transformers offer a range of models suitable for various tasks, with options like GPT-3 for advanced language understanding and generation.
Google's BERT: Bidirectional Encoder Representations from Transformers (BERT) by Google is another popular choice for natural language processing tasks, known for its bidirectional understanding of context.
Facebook's PyTorch-based models: Facebook's PyTorch framework supports various models for natural language processing, including BART (Bidirectional and Auto-Regressive Transformers) and RoBERTa (Robustly optimized BERT approach).
In a world increasingly reliant on AI-driven solutions, models like Microsoft's Phi-3-mini offer significant advancements in language understanding and reasoning capabilities. From enhancing communication platforms to powering innovative applications, such models pave the way for transformative technologies. However, responsible usage and thorough evaluation of such models are crucial to ensure ethical deployment and mitigate potential risks. By leveraging state-of-the-art AI models like the Phi-3-mini responsibly, developers can unlock new possibilities while prioritizing safety and fairness in AI-driven solutions.
For AI automation, adoption, and training for your employees, consider ExplainX's services. Contact us to explore how we can assist you in leveraging AI effectively.
Interlinked :
Kommentare